Long-Range Pose Estimation for Aerial Refueling Approaches Using Deep Neural Networks
Document Type
Article
Publication Date
2020
Abstract
Automated aerial refueling (AAR) provides unique challenges for computer vision systems. Aerial refueling maneuvers require high-precision low-variance pose estimates. The performance of two stereoscopic (stereo) vision systems is quantified in ground tests specially designed to mimic AAR. In this experiment, three-dimensional (3-D) pose-estimation errors of 6 cm on a target 30 m from the current vision system are achieved. Next, a novel computer vision pipeline to efficiently generate a 3-D point cloud of the target object using stereo vision that leverages a convolutional neural network (CNN) is proposed. Using the proposed approach, a high-fidelity 3-D point cloud with ultra-high-resolution imagery 11.3 times faster than previous approaches can be generated.
DOI
10.2514/1.I010842
Source Publication
Journal of Aerospace Information Systems
Recommended Citation
Lee, A., Dallmann, W., Nykl, S., Taylor, C., & Borghetti, B. (2020). Long-Range Pose Estimation for Aerial Refueling Approaches Using Deep Neural Networks. Journal of Aerospace Information Systems, 17(11), 634–646. https://doi.org/10.2514/1.I010842
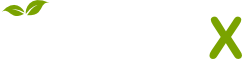
- Citations
- Patent Family Citations: 1
- Citation Indexes: 17
- Usage
- Abstract Views: 39
- Captures
- Readers: 21
Comments
The Link to Full Text on this page loads the PDF article as hosted at the AIAA portal, Aerospace Research Central.
This material is declared a work of the U.S. Government and is not subject to copyright protection in the United States. The material is in the public domain and can be reused without permission within the United States so long as the original source is acknowledged and fully cited.