Document Type
Article
Publication Date
9-9-2024
Abstract
A numerical method for evolving the nonlinear Schrödinger equation on a coarse spatial grid is developed. This trains a neural network to generate the optimal stencil weights to discretize the second derivative of solutions to the nonlinear Schrödinger equation. The neural network is embedded in a symmetric matrix to control the scheme’s eigenvalues, ensuring stability. The machine-learned method can outperform both its parent finite difference method and a Fourier spectral method. The trained scheme has the same asymptotic operation cost as its parent finite difference method after training. Unlike traditional methods, the performance depends on how close the initial data are to the training set.
DOI
10.3390/math12172784
Source Publication
Mathematics (e-ISSN 2227-7390)
Recommended Citation
Akers, B. F., & Williams, K. O. F. (2024). Coarse-Gridded Simulation of the Nonlinear Schrödinger Equation with Machine Learning. Mathematics, 12(17), 2784. https://doi.org/10.3390/math12172784
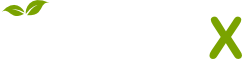
- Citations
- Citation Indexes: 1
- Usage
- Downloads: 41
- Abstract Views: 8
- Captures
- Readers: 2
- Mentions
- Blog Mentions: 1
- News Mentions: 1
Comments
© 2024 by the authors. Licensee MDPI, Basel, Switzerland.
This article is published by MDPI, licensed under a Creative Commons Attribution 4.0 International License (CC BY 4.0), which permits use, sharing, adaptation, distribution and reproduction in any medium or format, as long as you give appropriate credit to the original author(s) and the source, provide a link to the Creative Commons license, and indicate if changes were made.
Sourced from the published version of record cited below.