Document Type
Article
Publication Date
8-2019
Abstract
In recent years, convolutional neural networks have achieved state-of-the-art performance in a number of computer vision problems such as image classification. Prior research has shown that a transfer learning technique known as parameter fine-tuning wherein a network is pre-trained on a different dataset can boost the performance of these networks. However, the topic of identifying the best source dataset and learning strategy for a given target domain is largely unexplored. Thus, this research presents and evaluates various transfer learning methods for fine-grained image classification as well as the effect on ensemble networks. The results clearly demonstrate the effectiveness of parameter fine-tuning over random initialization. We find that training should not be reduced after transferring weights, larger, more similar networks tend to be the best source task, and parameter fine-tuning can often outperform randomly initialized ensembles. The experimental framework and findings will help to train models with improved accuracy.
DOI
10.1007/s00521-017-3285-0
Source Publication
Neural Computing and Applications (ISSN 0941-0643 | e-ISSN 1433-3058)
Recommended Citation
Becherer, N., Pecarina, J. M., Nykl, S. L., & Hopkinson, K. M. (2019). Improving Optimization of Convolutional Neural Networks through Parameter Fine-tuning. Neural Computing and Applications, 31(8), 3469–3479. https://doi.org/10.1007/s00521-017-3285-0
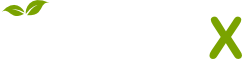
- Citations
- Citation Indexes: 46
- Patent Family Citations: 1
- Usage
- Downloads: 95
- Abstract Views: 46
- Captures
- Readers: 81
Comments
© 2019 The Authors.
This article is published by Springer, licensed under a Creative Commons Attribution 4.0 International License (CC BY 4.0), which permits use, sharing, adaptation, distribution and reproduction in any medium or format, as long as you give appropriate credit to the original author(s) and the source, provide a link to the Creative Commons license, and indicate if changes were made.
Sourced from the published version of record cited below.
Funding note: his work was sponsored by the Vehicles Directorate of the Air Force Research Laboratory.